Speaker 1: Dr. Vladislav Golyanik
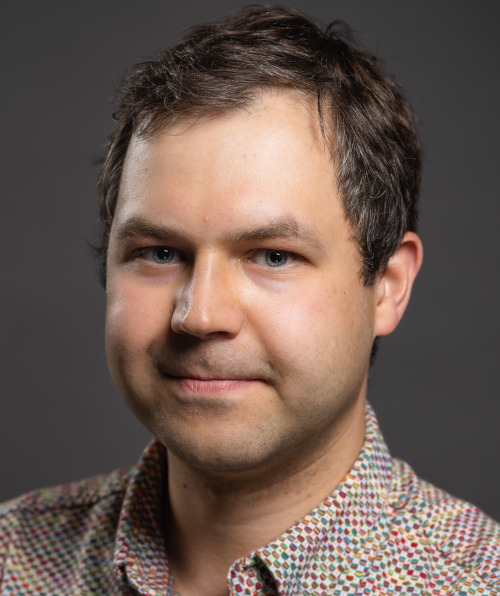
Vladislav Golyanik received a Ph.D. degree in computer science from the University of Kaiserslautern, Germany, in 2019 (advised by Didier Stricker). He is currently leading the 4D and Quantum Vision (4DQV) group at the MPI for Informatics. Before 4DQV, Vladislav was a Postdoc at MPI for Informatics (Christian Theobalt's group). He was also a visiting Fellow at NVIDIA, San José, CA, USA, and the Institute of Robotics and Industrial Informatics, Barcelona, Spain. Vladislav's team is interested in 3D reconstruction and neural rendering of rigid and non-rigid scenes, 3D generative models, quantum visual computing as well as physics-based methods in vision and graphics.
Speaker 2: Prof. Tali Treibitz
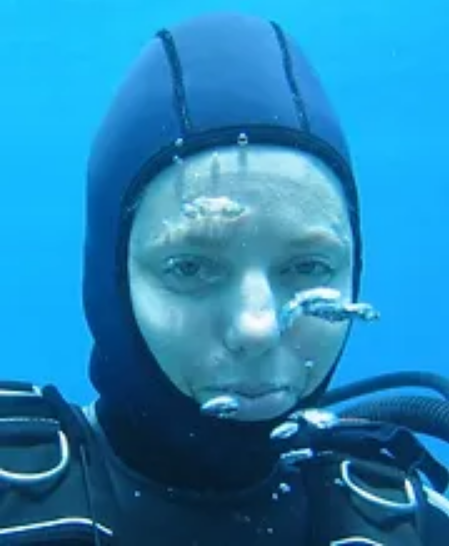
Prof. Tali Treibitz is the head of the Marine Imaging Lab, in the Department for Marine Technologies, Charney School of Marine Sciences, University of Haifa. Previously, she was a postdoc, working with Prof. David Kriegman in the Computer Vision group, Computer Science and Engineering department in the University of California, San Diego, and with Prof. Jules Jaffe in the Jaffe laboratory for Underwater Imaging in the Scripps Institution of Oceanography. Tali received her B.Sc. in Electrical Engineering from the Technion-Israel Institute of Technology and her M.Sc. in Electrical Engineering from Tel Aviv University. In 2010 she defended her Ph.D. entitled "Geometry and Photometry of Imaging Through a Medium" from the Electrical Engineering Technion, under the supervision of Prof. Yoav Schechner, in the Hybrid Imaging lab. Her research interests include computer vision and image processing with a focus on physics-based image restoration.
Title: Physics-Based Deep Learning for Underwater Image Restoration
Abstract:
Underwater image restoration is a challenging task because of strong water effects that increase dramatically with distance. This is worsened by lack of ground truth data of clean scenes without water. In CVPR 2018 we published a revised image formation model. We verified this model in extensive underwater experiments, and showed it is more accurate than the model commonly used with haze. The differences stem from the strong wavelength dependency underwater, which is much weaker in haze and fog. The model was followed the next year by an image restoration method (SeaThru) that used the revised model for restoring color in underwater images. In this talk I will show two new works that leverage the SeaThru model in deep learning methods. SeaThru-Nerf (CVPR 23) incorporated the revised image formation model in the NeRF framework, to demonstrate generation of novel underwater views, and more importantly, leveraged the NeRF representation to restore the underwater scenes, both the original ones and generate novel clean views. In Osmosis (under review), we combine the SeaThru model with guided diffusion, to demonstrate single-image recovery, including a depth map of the scene.